Advancements in healthcare machine learning are significantly transforming how medical services are delivered. The incorporation of sophisticated algorithms and AI-driven tools is enabling more accurate diagnoses and customized treatment plans, revolutionizing patient care. Healthcare is about to enter a new era thanks to machine learning, which is enabling individualized medication and early disease diagnosis.
The impact of machine learning isn’t limited to clinical settings alone. Administrative processes are also seeing a revolution, with AI systems helping to streamline operations, reduce costs, and improve efficiency. This technology is becoming increasingly indispensable, offering solutions to some of healthcare’s most persistent challenges.
The Role of Machine Learning in Disease Diagnosis
Machine learning algorithms are rapidly advancing the accuracy of disease diagnosis. These advanced tools help healthcare professionals identify diseases at earlier stages, which can significantly improve patient outcomes. For example, deep learning algorithms have shown promise in detecting conditions such as cancer and diabetic retinopathy with remarkable precision.
Traditional diagnostic techniques often rely heavily on the experience and expertise of the physician, which can lead to variability in results. In contrast, machine learning makes use of enormous volumes of data to find patterns that the human eye could overlook. This increases the accuracy of diagnoses and helps standardize the diagnostic process, making it more reliable.
Moreover, machine learning models can be trained on a variety of data sources, including medical records, imaging studies, and genomic data. This multi-faceted approach ensures a more comprehensive analysis, leading to better-informed decisions and, ultimately, better patient outcomes.
Enhancing Treatment Plans Through Predictive Analytics
Predictive analytics, powered by machine learning, is becoming crucial in formulating more effective treatment plans. These systems analyze large datasets to predict how patients will respond to different treatments, allowing doctors to customize therapies that are more likely to succeed. A study from Harvard Medical School highlights how predictive analytics can forecast patient recovery times and potential complications, leading to more efficient and personalized care.
The ability to predict patient outcomes accurately can be a game-changer in healthcare. For example, medical practitioners can identify patients who are at high risk of readmission by using predictive models, which enables them to intervene early and take preventive measures. This lowers the cost of hospital readmissions while simultaneously improving patient health.
Furthermore, predictive analytics can aid in the optimization of treatment protocols. By analyzing historical data, machine learning models can suggest modifications to existing treatment plans, making them more effective. By guaranteeing that every patient receives the best course of care, this individualized approach raises the standard of care overall.
Reducing Operational Inefficiencies
Operational inefficiencies in healthcare not only waste time and resources but also affect patient care quality. AI-powered tools are addressing these issues by optimizing scheduling, streamlining administrative tasks, and improving patient flow management. For instance, hospitals are now using ML models to predict patient admission rates, which helps in better resource allocation and reduced waiting times.
Administrative duties can be labor-intensive and prone to mistakes, including making appointments, billing, and managing inventory. These procedures can be automated by machine learning algorithms, which lowers the possibility of mistakes and frees up workers to concentrate on more important work. This boosts the entire patient experience in addition to increasing efficiency.
Additionally, machine learning can assist in optimizing resource utilization. For example, predictive models can forecast the demand for medical supplies, ensuring that hospitals are well-stocked and prepared for any situation. This proactive approach helps minimize wastage and ensure that resources are used effectively.
Ethical Considerations and Data Privacy
As machine learning integrates deeper into healthcare, ethical considerations, and data privacy, concerns come to the forefront. Ensuring patient data is secure and used ethically is paramount. Healthcare providers and developers must adhere to strict regulations and ethical guidelines to maintain patient trust and data integrity. Efforts to develop transparent algorithms and ensure clinical explainability are ongoing challenges in the industry.
The use of machine learning in healthcare involves handling vast amounts of sensitive patient data. Ensuring the privacy and security of this data is critical to maintaining patient trust. Healthcare organizations must implement robust data protection measures and comply with regulations such as the Health Insurance Portability and Accountability Act (HIPAA).
In addition, ethical issues need to be taken into account when creating and using AI systems. Algorithms, for example, ought to be created with the least amount of prejudice and to guarantee impartiality when making decisions. In order to foster trust and guarantee that medical personnel can comprehend and verify the advice provided by these systems, transparent and explicable AI models are crucial.
The Importance of Interdisciplinary Collaboration
Continued advancements in machine learning in healthcare are largely dependent on interdisciplinary collaboration. Bringing together experts from fields such as medicine, data science, and bioinformatics allows for more innovative solutions and robust applications. This combined expertise can address complex challenges and accelerate the development of groundbreaking healthcare technologies.
Interdisciplinary collaboration fosters a holistic approach to problem-solving. Medical professionals provide insights into clinical practice, data scientists contribute analytical expertise, and bioinformaticians offer a deep understanding of biological data. This collaborative effort ensures that machine learning models are developed with a comprehensive understanding of both the technical and clinical aspects of healthcare.
Collaboration can also result in the creation of fresh research approaches and the revelation of fresh discoveries. By combining diverse perspectives, interdisciplinary teams can identify unique opportunities and develop innovative solutions that may not be possible within a single discipline. This collaborative approach is essential for driving continued progress in the field of healthcare machine learning.
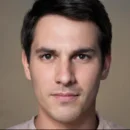
Robert Wiley is a versatile and accomplished writer with expertise spanning multiple niches, delivering insightful and engaging content across various fields. His diverse experience and deep knowledge make him a sought-after author in the world of digital writing.